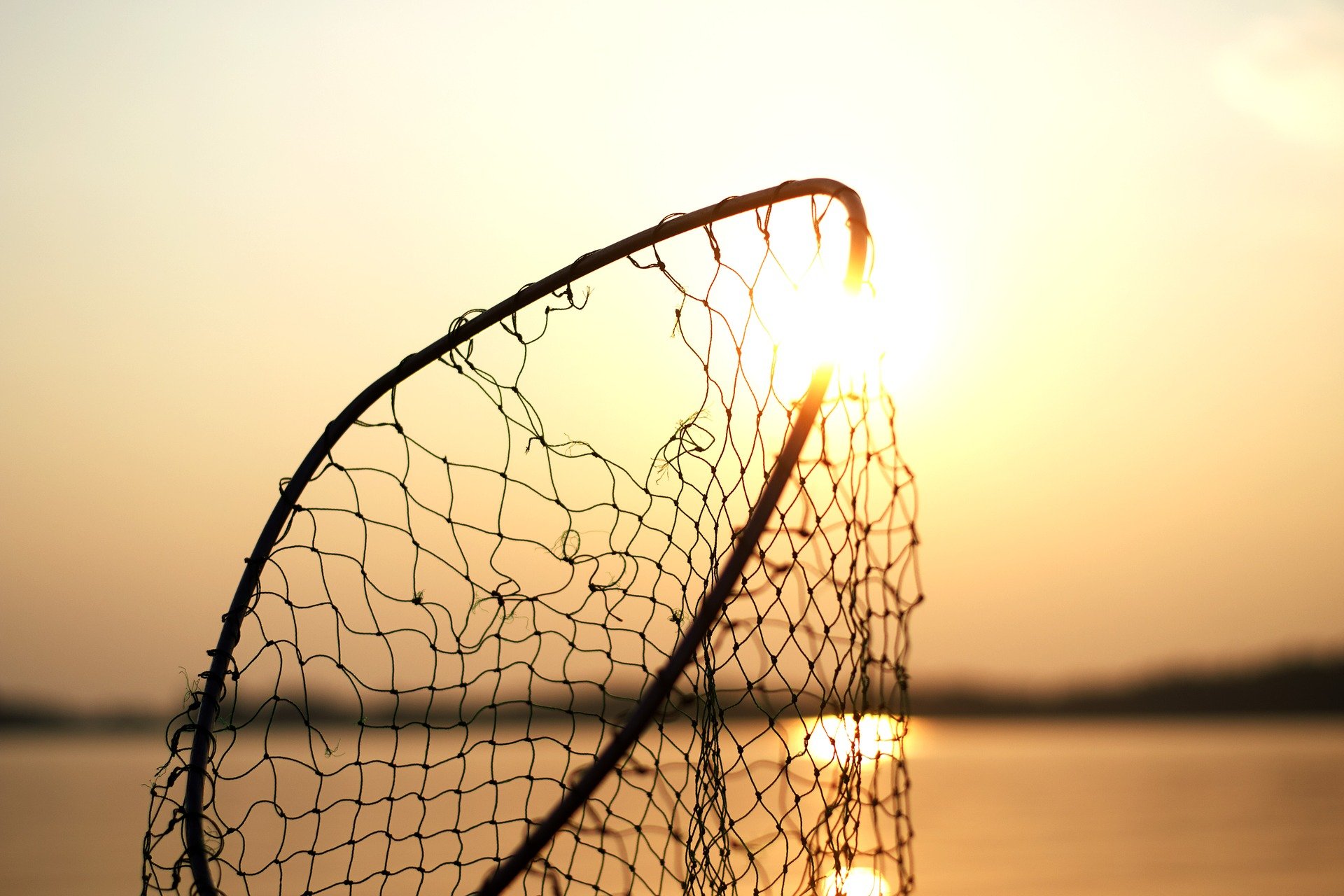
Beware the lure of big effect
A few weeks ago, XKCD, a “webcomic of romance, sarcasm, math, and language”, posted a comic showing infection rates over time for a vaccinated cohort compared to a control group. The graph (which is hand-drawn, as is the comic’s style), serves to highlight the message that the vaccines that have been developed so far to fight COVID-19 are so effective that no statistical analysis is needed to be confident that they work. Indeed, the webcomic’s caption says “statistics tip: always try to get data that’s good enough that you don’t need to do statistics on it”.
I am a huge fan of XKCD, and of its author Randall Munro. But I find something about this comic in particular discomfiting, from the perspective of a social scientist.
First of all, when Munro talks about good data, he seems to mean good effect sizes. This is a small point, but the reason the vaccine results are so easy to see isn’t because they have high quality data – the results could be described in two columns in Excel, each containing only ones and zeros. Instead, it’s because the vaccines are so effective.
That aside, there’s a real hazard in expecting, or hoping for, large effect sizes. Vaccine trials can produce statistically significant results in small samples, because of the power of their effects. As we’ve seen on Twitter over the past few months, studies of mask wearing, which leads to more modest effects on modest subsamples, even when people comply with their treatment assignment, do not produce such statistically stark results. This isn’t a matter of ‘data quality’ – it’s a matter of the type of study (an encouragement design), and the mechanism of the intervention. If we expect large effects, then we design our studies differently, and we end up being disappointed by reducing infections by a ‘mere’ 14%.
We have seen this same phenomenon across other domains in the social sciences, where effects are, by and large, small. We can think about this like fishing with a net. Our sample size, and study design, determine the size of the holes in the net – the bigger the holes, the larger the effect that we need to see. For too long, we’ve gone fishing with shark-sized nets, and erroneously concluded that there are no fish in the sea – in fact, there could be lots, which we simply fail to catch. Anything that does get caught is either a shark, or an unlucky smaller fish, caught in just the right place.
This has been the story in education, where we spent a decade believing the effect sizes were on average 8 times larger than they really were; it’s the case in social psychology, where small sample sizes combined with publication biases produced evidence of large but false effects. It’s a risk that we can all fall into; the incentives of the experimental designer run towards hoping for larger effects – not only are they cheaper to run, it feeds our natural optimism bias.
This bias is seductive – we want to believe in silver bullets, or interventions that can, single handedly, change the world. But in social policy, such things are rare, or even non-existent. However, a commitment to evidence-based policy means a commitment to trying to overcome these biases, and seeing things as they are.
Dr Michael Sanders is Chief Executive of What Works for Children’s Social Care, and a Reader in Public Policy at the Policy Institute, King’s College London.